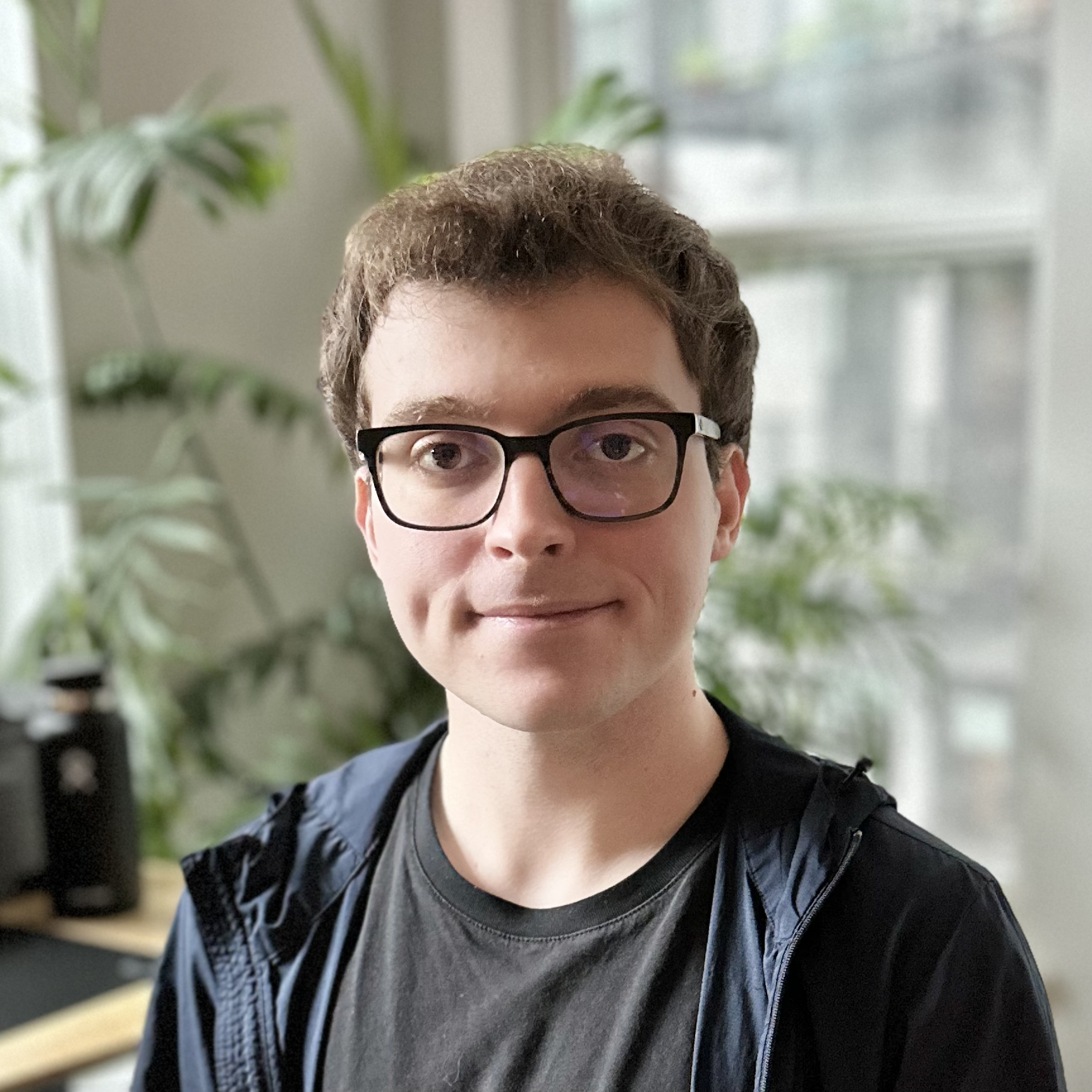
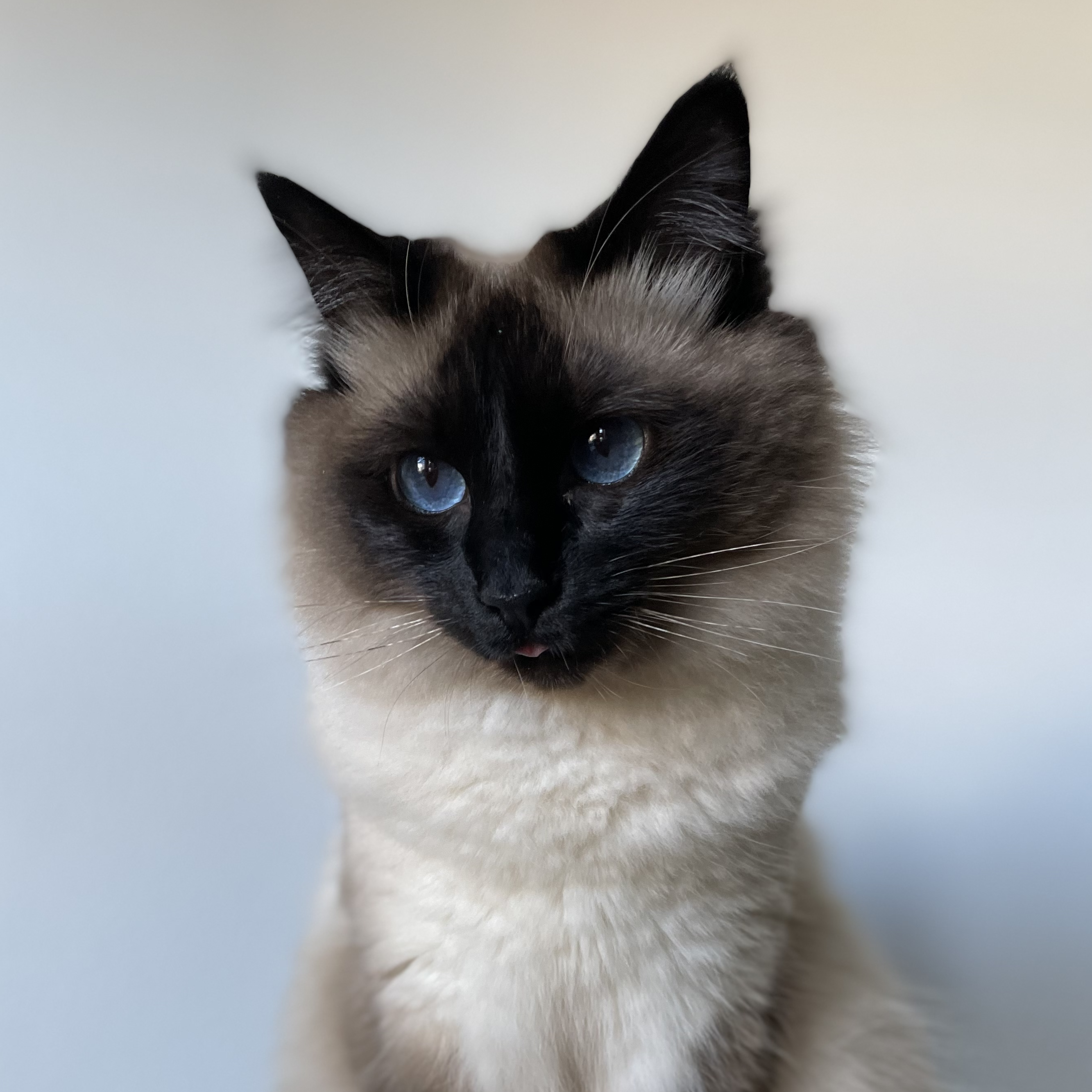
Nicholas Tomlin
PhD Student
Berkeley EECS
nicholas_tomlin@berkeley.edu
I'm a fifth-year PhD student in Berkeley EECS, where I am advised by Dan Klein and affiliated with Berkeley NLP and BAIR. I am also a part-time researcher at Microsoft Semantic Machines, where I work with Jason Eisner and Jacob Andreas. I was previously funded by the NSF Graduate Research Fellowship Program.
Before coming to Berkeley, I was an undergrad at Brown University, where I majored in math and linguistics and was advised by Ellie Pavlick.
My research focuses on natural language processing, with an emphasis on building systems that can efficiently collaborate with humans via language in rich grounded environments. I have worked on:
-
Pragmatic dialogue agents
I'm interested in enabling language models to collaborate effectively and efficiently with humans, through the use of external tools and pragmatic reasoning. We released a new class of tasks known as decision-oriented dialogue, which is an extension of task-oriented dialogue to settings in which humans and computers must collaborate in order to solve challenging optimization problems. We also wrote a survey paper on pragmatic phenomena, tasks, and modeling approaches in language grounding.
-
Grounding language into abstractions
In order to understand and communicate about the real world, language models must be grounded. However, language grounding involves not just mapping language to visual percepts, but also understanding language about abstract concepts, actions, and plans. We collected a dataset of language about the game of Go and analyzed the extent to which abstract concepts are encoded in the intermediate state representations of models like AlphaGo.
-
Detecting LLM-generated text
We built Ghostbuster, the world's best supervised detector for LLM-generated text. It works by passing documents through a series of weaker language models, running a structured search over combinations of features from these models, and then training a linear classifier on the extracted features.
-
Computer crossword solving
We built the Berkeley Crossword Solver, a state-of-the-art system for solving American-style crosswords based on dense passage retrieval, belief propagation, and local search. In conjunction with Dr.Fill, our system was the first computer program to outscore all humans at the American Crossword Puzzle Tournament and was featured in Discover, Wired, New Scientist, Slate, and the BBC. I also co-wrote a pop article about the linguistics of crosswords for The Atlantic.