Generative Models 001
One view of machine learning is that it is the study of algorithms that
make inference about probability distributions. In this view, the dataset
is viewed as a sample from some underlying unknown probability distribution.
That is for some unknown probability distribution
- Density estimation: Given a dataset
, estimate the probability density function of the underlying distribution . - Data generation : Given a dataset
, generate new samples from the underlying distribution . - Representation Learning : Given a dataset
, learn a representation of the underlying distribution . This is particularly useful for high-dimensional support we have limited annotated data.
Generative Models
We focus on three classes of generative models:
- Likelihood-based models Given a family of parametric models
, we aim to learn a model from the family that is close to the true distribution . This is done by maximizing the likelihood of the observed data under the model, i.e. - Implicit models An alternative approach to representing a probability distribution, is to
model
the generative process. In other words, instead of explicitly modeling the density, we learn how to
directly sample new points
. Note that with enough samples, we can estimate the density of the distribution by computing the empirical distribution . - Diffusion models An emerging class of generative models is
or models. Note that in likelihood-based models we care about that informs us about the direction of descent in the parameter space. In diffusion models, we instead care about the that informs us about how the inputs themselves should be perturbed to increase the probability of the output. Additionally, diffusion models are typically samplers, where the inputs are perturbed multiple times to generate an output.
Unsupervised Representation Learning
Consider the setting where you have access to large amounts of unlabelled images from the internet,
Contractive Autoencoder
One approach is performing non-linear dimensionality reduction using neural networks. For example,
with a
Denoising Autoencoder
As we've seen in lecture, data augmentations present a powerful tool for improving the generalization of
neural networks. An instantiation of this in the autoencoder setting is the denoising autoencoder
Extracting
and Composing Robust Features with Denoising Autoencoder, Vincent et al 2008
. In this setting, we corrupt the input
Comparing Distributions
One of the core objectives of generative models is to learn
- Kullback-Leibler Divergence:
- Jensen-Shannon Divergence:
, where
Note that the KL divergence is generally asymmetric, i.e.
Variational Autoencoder
VAEs fall in the class of likelihood-based models. The goal is to learn a model
Evidence Lower Bound
Given a latent-variable model,
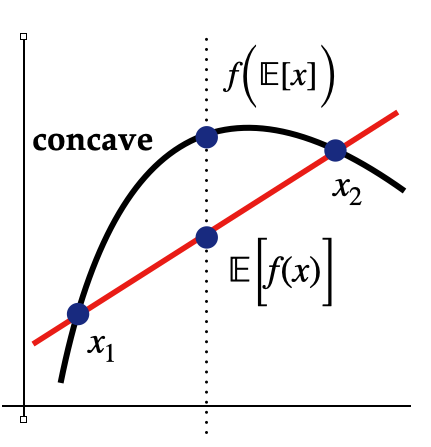
In particular, for any concave function (

Gradient based optimization of the above formulation is still difficult, since taking gradient
through a stochastic node (e.g. sampling from a distribution) is not differentiable. To overcome
this, we use the reparameterization trick to sample from the posterior distribution
Generative Adversarial Networks
As an implicit model, GANs present a different perspective to modelling the data distribution Generative Adversarial Networks , Goodfellow 2014. In particular, GANs are composed of two networks:
- Generator:
, where - Discriminator:
, where

Training
The high level intuition behind training GANs is that we want to train the generator to create samples
that
are
indistinguishable from the real data. At the same time, we want to train the discriminator to be able to
distinguish between real and fake data. This presents the following
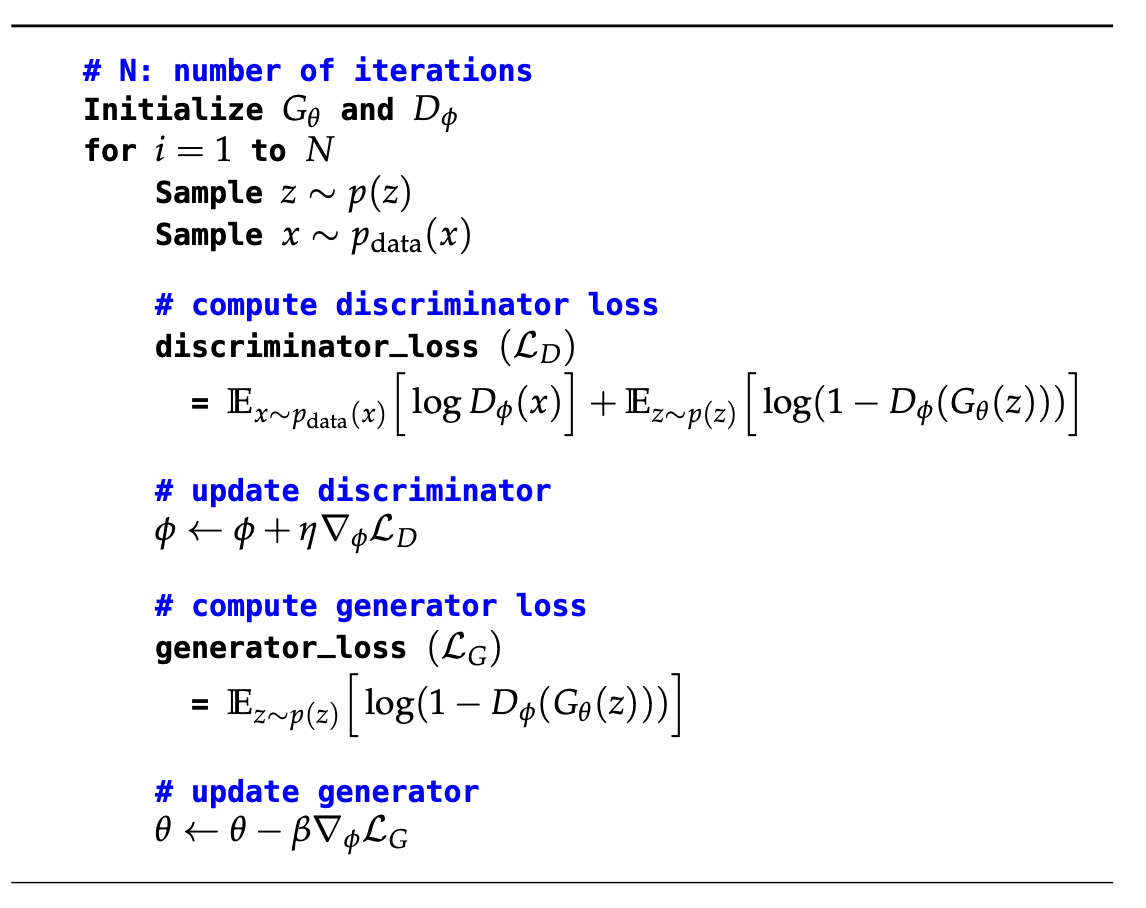
Sampling
Sampling from GAN is done by sampling latents from the prior distribution
Diffusion Models
The algorithms and models we've considered directly learn to either estimate density (VAEs) or generate samples (GANs). Notably, these algorithms are single-step, meaning that there is a single step of probabilistic inference (variational or implicit).
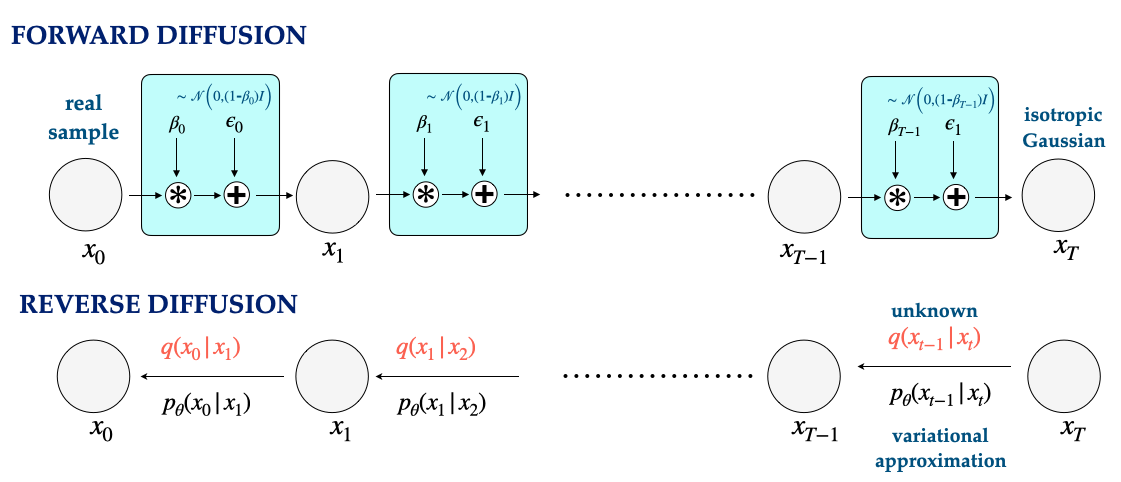
Diffusion models are a class of generative models that are
- Forward: In this phase, we start from samples from the real distribution and iteratively add
noise
to the samples. In particular, starting from
we have: Notably, the variance of the distribution is a function of the step , and the variance increases as we move forward in time. A typical example Denoising Diffusion Probabilistic Models, Ho 2020 of this would be linearly increasing from to . - Reverse: Sampling from the diffusion model is done by starting from a sample from our
target
distribution (e.g. isotropic Gaussian) and iteratively denoising the noisy inputs. In the Markov
chain
illustrated above this corresponds to starting at
and moving backwards in time, such that is the denoised sample. Performing this denoising step, however requires us to estimate which is not tractable. Instead, we perform a variational inference to estimate this posterior distribution, and maximize the ELBO (as in VAEs) for each time-step. A key insight that enables such inference, is that one can recover a closed form Denoising diffusion probabilistic models expression for .
For more details on the diffusion model, we refer the reader to these wonderful blog posts Generative Modeling by Estimating Gradients of the Data Distribution, Song 2021 What are Diffusion Models?, Weng 2021.
Training
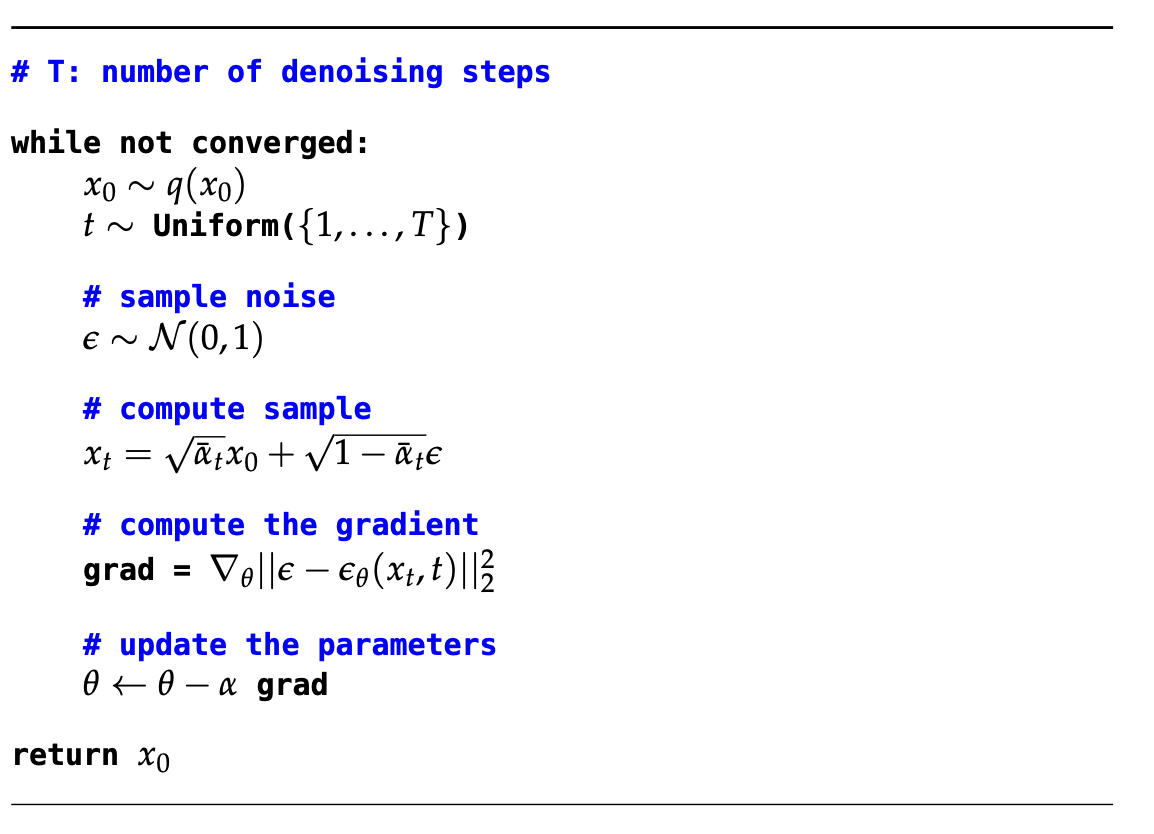
With a trained estimator for the score function, we can reverse the diffusion process to sample from the data-distribution.
